trending
12 Key AI Concepts Explained: LLMs, RAG, Prompt Engineering & More
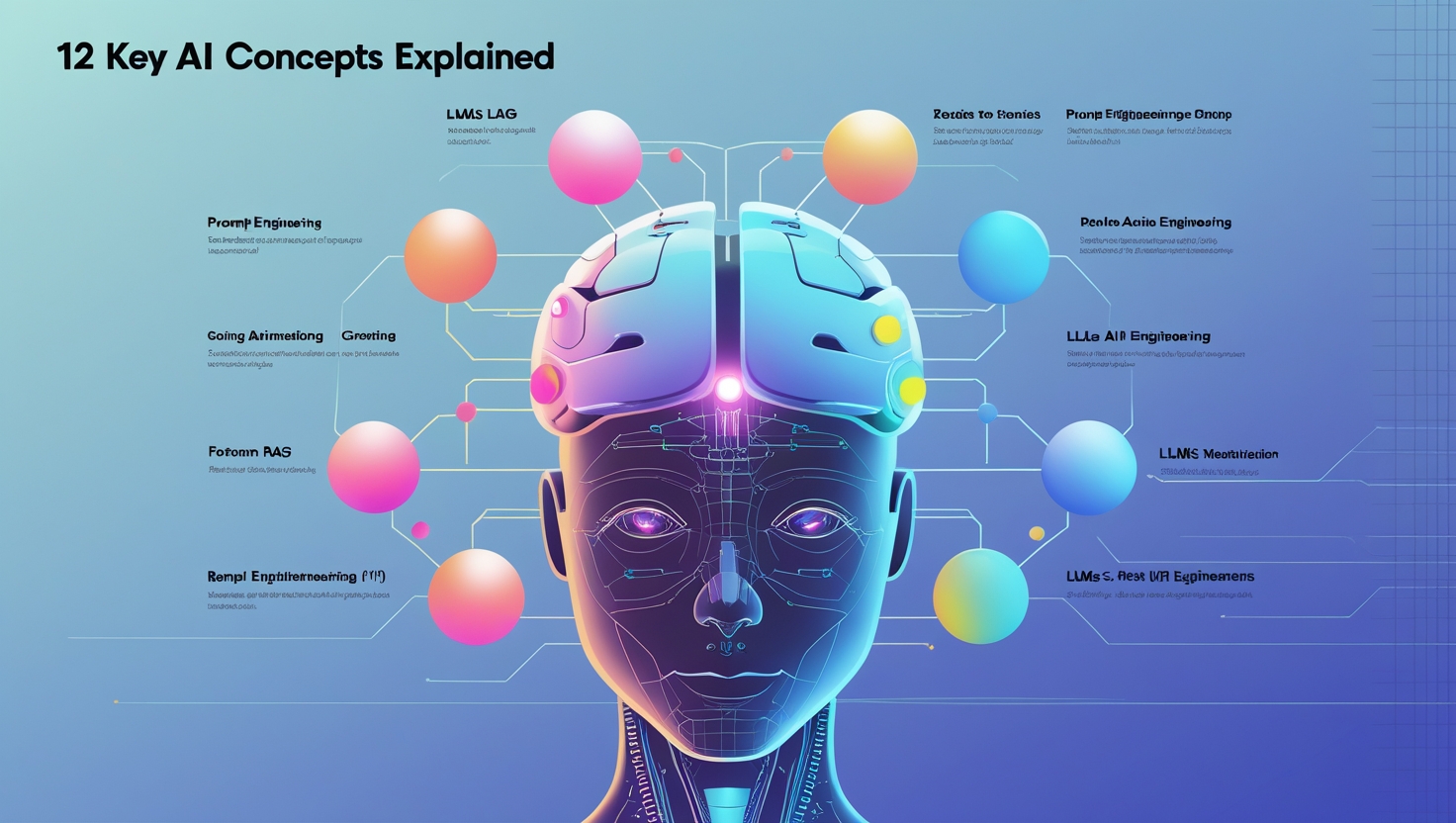
A vital comprehension of artificial technologies and their related concepts is essential in the ever changing world of AI developments. Now, let us look into 12 core concepts of AI which are blazing a trail into the future.
- LLM (Large Language Model): AI technologies have LLMs like GPT-4, Claude, and Gemini enabling them to understand and generate text. These models are crucial in the development and use of chatbots, coding assistants, and content creation applications, where machines understanding and articulating human language is done with superb precision.
- Transformers: AI models understanding the meaning, relations and order of words stem from modern day transformers technology. There has been a shift in the relation between humans and machines with the introduciton of BERT and GPT where interacting using machines is far easier because of the intuitive language model.
- Prompt Engineering: Instructions must be very specific if accurate results are to be drawn from AI systems. The use of system prompts, step guides, and safety prompts increases the quality of answers an AI gives.
- Fine Tuning: Meeting industry specific standards is now possible with AI models created for legal and medical tasks through process of training an AI model on datasets which specialize in such tasks.
- Embeddings: As one of the most important technologies in understanding relationships between words or documents, embeddings enhance the relevance and accuracy of search engines and recommendation systems.
- RAG (Retrieval-Augmented Generation): Establishing a balance between retrieving information and generating content, RAG incorporates databases with generative systems in a way that ensures creativity blended with factual accuracy.
- Tokens: Resource allocation optimization begins with token management which directly affects the cost and performance of extracting AI capabilities, making the efficient structuring of text chunks crucial.
- Hallucination: The reliability of AIs in repeating human input information is compromised when incorrect or fabricated responses are generated; rigorous fact-checking and better strategies lighten the burden.
- Zero-Shot Learning: This feature enables AIs to perform disparate tasks effortlessly without prior training on specific data sets, reducing time expenditure and broadening application versatility.
- Chain-of-Thought Reasoning: Explaining the process behind reasoning in complex situations, such as in multi-step problems, increases the transparency of the today AI-enabled systems decision-making processes.
- Context Window: The amount of information that can be seen simultaneously with one glance impacts how well AI systems process long texts and dialogues; broad windows with no restrictions improve interactions over prolonged periods.
- Temperature Settings: These modifications influence the level of ingenuity or precision applied. Lower values, for instance, ensure dependable output, while high values allow more subjective interpretation, thus providing more freedom to AI assistants.
Anticipating further changes: the integration of text, images, and audio will be combined with smaller and faster models that prioritize safety and ethical guidelines from the Constitution. This will all come together, perhaps, guiding us toward fully autonomous agents capable of completing entire assigned tasks without any human assistance.
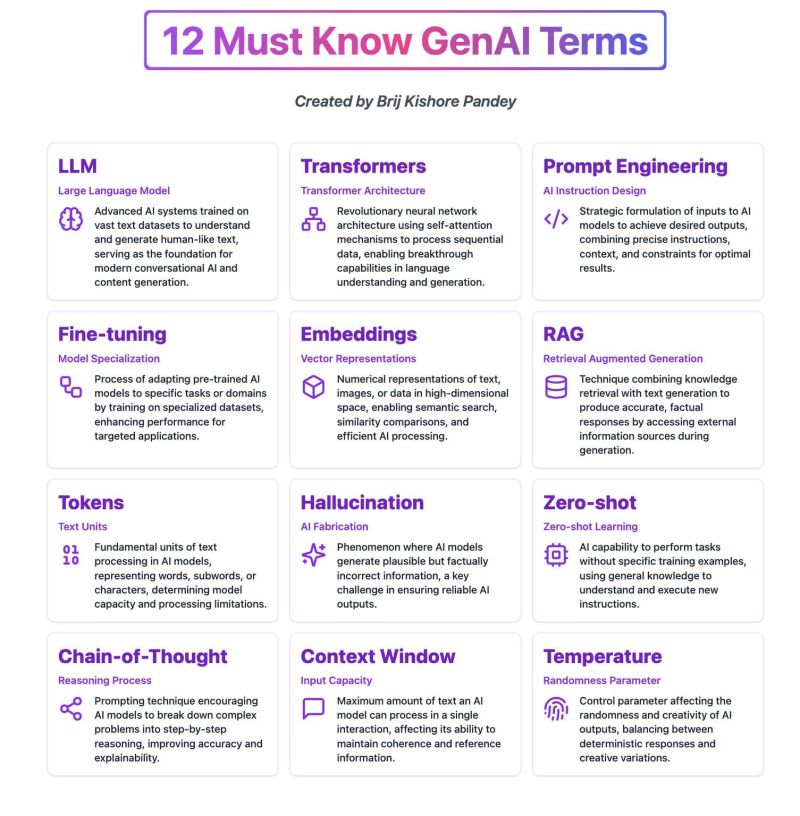
Outstanding